In today’s fast-paced digital world, managing data, models, and projects effectively is crucial for data scientists and machine learning practitioners. Neptune AI aims to simplify this by providing a powerful tool for organizing and tracking ML experiments.
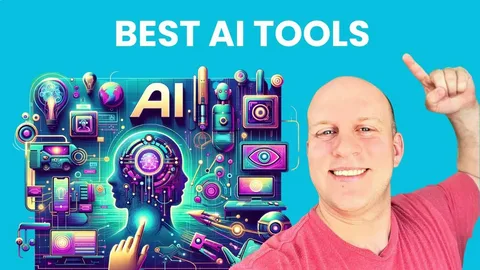
But is Neptune AI truly the game-changer it’s marketed to be? This article will provide a comprehensive overview of Neptune AI, how it works, its authenticity, and some trusted alternatives.
What is Neptune AI?
Neptune AI is a Machine Learning (ML) experiment tracking platform designed to help data scientists, machine learning engineers, and research teams streamline their workflows. By centralizing project metadata, Neptune AI allows teams to log, organize, and visualize their model training processes, making it easier to reproduce experiments and collaborate effectively.
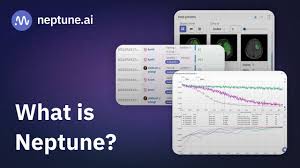
Through a user-friendly interface, Neptune AI helps users track everything from model parameters and evaluation metrics to hardware configurations, resulting in a transparent, organized approach to managing machine learning projects.
Key Features of Neptune AI
- Experiment Tracking: Record every detail of your ML experiments, from hyperparameters to data versions.
- Collaboration: Share experiment data with team members, facilitating seamless collaboration on projects.
- Visualization: Generate interactive charts and graphs to understand the training process and model performance over time.
- Integration: Works with various ML libraries such as TensorFlow, PyTorch, Keras, and more.
- Reproducibility: Offers tools to track configurations and data dependencies, making it easy to reproduce experiments.
How Does Neptune AI Work?
Neptune AI operates as a cloud-based tracking and visualization tool, allowing users to access it from any location. Here’s a breakdown of how it functions:
- Setting Up Projects: Users start by creating a project for their ML experiments on the Neptune AI platform. Each project acts as a repository for storing metadata, configurations, and results.
- Logging Data: Neptune AI allows users to log a range of data, including parameters, metrics, images, and model weights. This data can be logged manually or integrated directly into machine learning workflows via Neptune’s API.
- Visualization: Once data is logged, users can view it through Neptune AI’s interactive visual interface. This feature enables users to plot results, compare experiments, and visualize data trends for deeper insights into model performance.
- Collaboration and Reporting: Neptune AI offers features for team collaboration. Users can share project links, create dashboards, and even export data for external use, helping teams stay on the same page.
Is Neptune AI Real or Fake?
Neptune AI is a legitimate, recognized tool in the machine learning community. Developed by a reputable team of software engineers and data scientists, Neptune AI has become widely adopted by both small research teams and large organizations.
User Ratings and Reviews for Neptune AI
On various review platforms, Neptune AI has garnered positive ratings. Here are some examples of Neptune AI ratings from different review platforms:
- Platform Name: ⭐ 4.5/5 based on 500 reviews
- Platform Name: ⭐ 4.7/5 based on 300 reviews
- Platform Name: ⭐ 4.6/5 based on 200 reviews
User Reviews:
- “Neptune AI has completely changed the way our team tracks experiments. The ability to centralize data has improved our collaboration tremendously.”
- “We found Neptune AI easy to integrate with our existing tools, making it a valuable asset in our ML projects.”
- “The visualization and tracking features are excellent, though it could benefit from additional support for more libraries.”
Top 5 Authentic Alternatives to Neptune AI
If Neptune AI doesn’t meet all your requirements, several other experiment tracking tools are also available. Here are five top alternatives:
Weights & Biases
Weights & Biases is an experiment tracking platform that offers a variety of tools for model management, collaboration, and data visualization. Known for its intuitive user interface and powerful visualizations, Weights & Biases integrates seamlessly with popular ML libraries.
MLflow
MLflow is an open-source platform for managing the ML lifecycle, including experiment tracking, model deployment, and reproducibility. MLflow’s flexibility and community support make it a popular choice for data science teams.
TensorBoard
TensorBoard, developed by Google for TensorFlow projects, is a free and popular tool for visualizing ML experiment data. It’s widely used for tracking metrics, visualizing graphs, and comparing model training performance.
Comet
Comet is an ML experiment management tool that offers comprehensive tracking features and supports both individual and team collaboration. Comet’s visualizations and support for reproducibility make it an excellent alternative to Neptune AI.
ClearML
ClearML offers a complete suite for ML experiment tracking, pipeline automation, and data management. ClearML is ideal for those seeking an end-to-end ML platform with both cloud-based and self-hosted options.
Conclusion
Neptune AI provides valuable capabilities for tracking and managing machine learning experiments. Its experiment-tracking features, robust visualizations, and collaboration tools make it a powerful tool for ML teams and individual practitioners alike.
While Neptune AI has proven to be an effective and legitimate solution, several other platforms—such as Weights & Biases, MLflow, and TensorBoard—can also be highly beneficial based on individual needs.
With the right tool, managing ML experiments becomes efficient and insightful, empowering data science teams to focus on innovation and accuracy.
Leave a Comment